What are the Limitations of Artificial Intelligence?
Updated: Nov 28, 2024
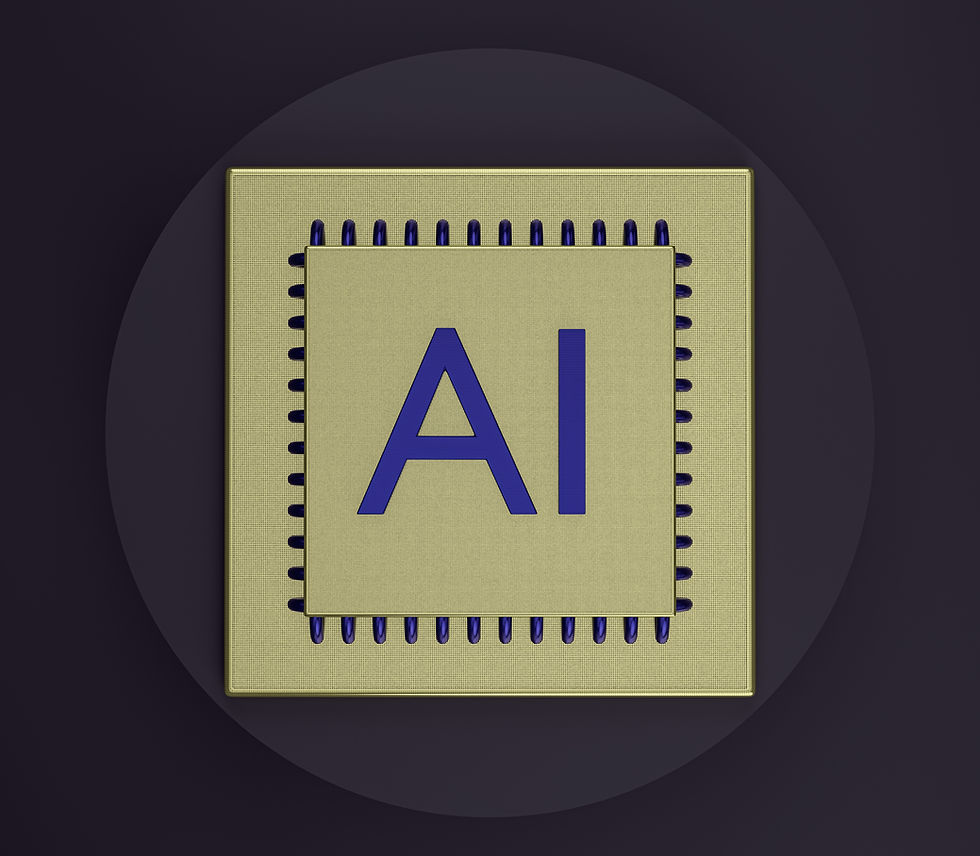
Artificial intelligence (AI) has made incredible advances in recent years, but the technology still has some key limitations (Read: How to Use Artificial Intelligence to Save Time on Daily Tasks).
Although AI can automate tasks, create content ( See 7 AI Copywriters to Help You Create Better Content), generate text, and even beat humans at games like chess and Go, it does have restrictions in what it can accomplish.
Understanding the current limitations of artificial intelligence is important as we integrate these systems into more aspects of our lives.
What are the Limitations of Artificial Intelligence?
Artificial intelligence (AI) has enormous potential to transform our lives. But the technology also has significant limitations and weaknesses that must be considered.
This article explores the most notable current restrictions and pitfalls of AI that users and developers need to keep in mind.
1. Narrow Capabilities
One of the biggest limits of today's AI is that it's narrow or weak AI, not general AI. Current systems only have intelligence focused on accomplishing specific, narrow tasks.
For example, an AI may be trained to generate product descriptions or chat with customers, but it lacks generalized reasoning skills. Unlike humans who have broad intelligence, today's AIs are specialized for their role.
This narrow scope restricts how helpful AI can be on tasks outside its speciality. Expecting a sales AI to suddenly pivot to medical analysis will fail. Broad problem-solving requires general AI.
2. Limited Memory and Knowledge
Humans accumulate vast memory and knowledge that aids our reasoning and decision-making. In contrast, AI systems' memory is restricted to data they've been specifically trained on.
This hinders the ability to apply learned knowledge in new situations the way people can. Without robust memory, AIs cannot link related concepts or adapt knowledge flexibly. Expanding training data is the only solution, which is costly.
3. Lack of Common Sense
Common sense encompasses the countless informal rules and intuitions people subconsciously use to function. This important context is utterly missing from today's AI.
For example, an AI told to organize chairs might stack them in a literal pile rather than arrange them logically for humans to sit. Without common sense, AIs easily misinterpret situations.
No one formally teaches people common sense, we intuit it from the world. Finding ways to ingrain common sense remains an unsolved AI challenge.
4. Inability to Generalize
A related limitation is that current AI systems cannot generalize knowledge beyond their training data. Feed an AI 1,000 dog photos and it will learn to recognize dogs. But feed it just one cat photo and it cannot apply prior learning to identify this new animal.
This inability to generalize from limited examples makes AI systems brittle. They require huge training datasets covering all scenarios, which is not always feasible. AI lacks inherent adaptability.
5. Lack of Transparency
The inner workings of AI systems are largely black boxes, even to their creators. With techniques like deep learning, the system logic that generates decisions and predictions is not understandable by humans.
This lack of transparency means it can be difficult to diagnose why an AI made a certain choice or result. Without explainability, it's hard to troubleshoot errors or definitively understand AI behaviour.
6. Vulnerable to Bias and Errors
Since AIs rely on training data, they inherently absorb any biases, errors, or flawed assumptions contained in that data. This can lead to prejudiced and unethical results.
Careful data preparation and debiasing methods are required to prevent encoding biases in AI systems. And training data must encompass enough diversity and breadth to avoid narrow perspectives.
7. Data Dependency
Today's data-driven AI techniques are heavily dependent on massive training datasets relevant to their task.
Gathering, cleaning and preprocessing these datasets requires substantial human effort and expense.
Many AI projects fail simply because sufficiently large, high-quality training data is lacking. Even for mundane tasks and vast data may be needed to train an accurate AI. This data bottleneck hinders progress.
8. Catastrophic Forgetting
When new data is used to train an existing AI system, it often catastrophically forgets previous learning, overriding it entirely with the new data. Continually expanding an AI's skills becomes challenging.
Retraining a face recognition AI with new faces may erase its prior facial knowledge completely. Rather than building incrementally, training sets often conflict. New techniques that allow additive learning without losing past knowledge remain scarce.
9. Inability to Transfer Learn
Unlike people, narrow AI systems cannot readily apply knowledge or skills learned for one task to a related but slightly different task through transfer learning.
For example, a company wants to pivot to a chatbot created for customer service to instead handle product education.
The entire system will need retraining, unable to transfer prior conversation skills to this new domain.
This makes adapting AIs to new uses extremely inefficient compared to flexible human learning. Transfer learning capability is an active area of AI research.
10. Lack of Common Sense
Common sense encompasses the countless informal rules and intuitions people subconsciously use to function. This important context is utterly missing from today's AI.
For example, an AI told to organize chairs might stack them in a literal pile rather than arrange them logically for humans to sit. Without common sense, AIs easily misinterpret situations.
No one formally teaches people common sense, we intuit it from the world. Finding ways to ingrain common sense remains an unsolved AI challenge.
11. Brittle Performance
Many AI systems are brittle, meaning minor changes to inputs or the environment can cause catastrophic failures. Researchers intentionally design corner case examples called adversarial attacks that easily trick AI.
For instance, distorting an image imperceptibly leads computer vision systems to misidentify objects completely. This sensitivity to small perturbations reveals the inherent fragility and lack of adaptability of today's AI algorithms.
12. Computational Expensiveness
Modern AI often relies on compute-intensive neural networks with millions of parameters trained using vast amounts of data. This necessitates expensive high-end hardware and lengthy training processes consuming extensive energy.
The costs of computational resources and energy for developing and running AI systems can be prohibitive for many organizations. More efficient methods are needed to make AI scalable and sustainable long term.
13. Lack of Reasoning Capabilities
Unlike human thinking, most AI systems today do not perform cognitive activities like reasoning, planning, and logic. Instead, they function through statistical pattern recognition within data.
This limits their ability to operate rationally, explain conclusions, incorporate conceptual knowledge, and handle complex challenges requiring reasoning chops beyond their training data.
14. Difficulty Performing Open-Ended Tasks
Today's AIs excel at well-defined tasks with clear objectives like labeling images or translating text. However, they struggle with open-ended tasks that lack formal constraints and goals.
Activities requiring holistic situational awareness, customization, and complex improvisation exceed current AI capabilities. The unstructured nature of such tasks remains an ongoing challenge.
15. Inability to Understand Language Nuances
Despite advances in natural language, today's AI cannot fully comprehend nuances of human communication requiring deeper understanding. The irony, humour, sarcasm and cultural references frequently mislead AI.
This forces reliance on simplistic language, limiting the AI's conversational capabilities. Until more human-like language understanding is achieved, faulty interpretations and responses will persist.
16. Lack of General World Models
People have expansive mental models of the world based on accumulated common sense and experience. AI systems lack analogous general world models for navigating novel environments and situations.
With narrow training, AIs cannot wing it and improvise like humans when interacting with unfamiliar scenarios. This hinders versatility and adaptability. Developing rich world models remains difficult.
SEE MORE AI TOOLS YOU SHOULD BE USING
Risks and Dangers Associated with AI's Limitations
Given these known limits of artificial intelligence, employing AI irresponsibly brings significant risks if its abilities are overestimated. Some notable dangers include:
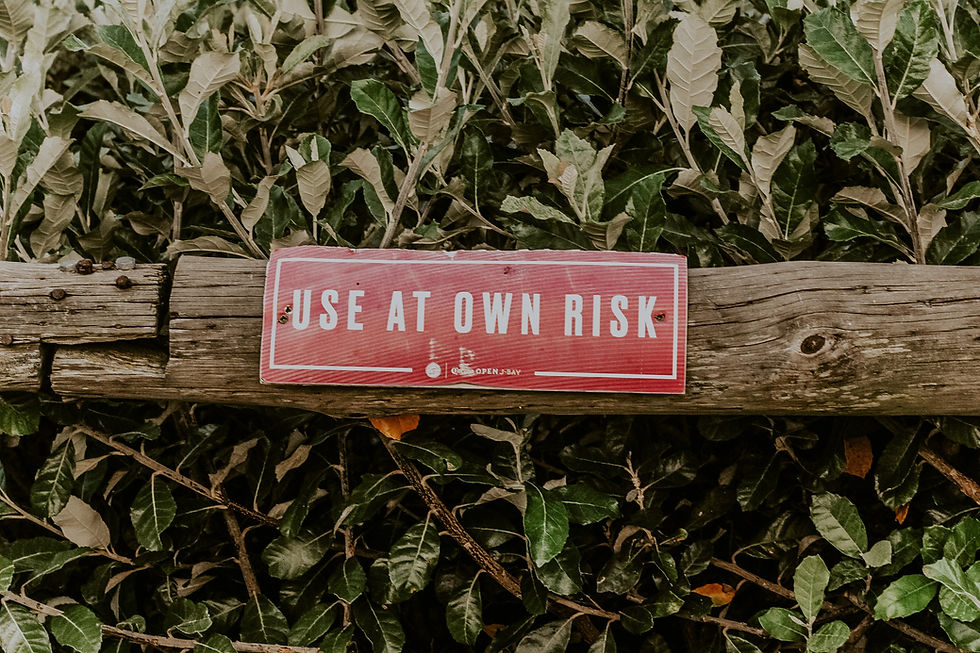
Faulty Automated Decisions
Due to brittleness and lack of common sense, AI systems will make incorrect or unfair judgments when used for automated decisions affecting people's lives, like loan approvals. Accountability for harmful AI decisions also becomes unclear.
Security Vulnerabilities
The inability of AI systems to adapt to unforeseen situations and adversarial attacks leaves them open to cybersecurity manipulations that human judgment could override. The lack of transparency amplifies this.
Loss of Privacy
Vast data collection coupled with limited explainability of how that data gets used poses privacy dangers. Who has access to user data and how it trains AI algorithms should have clear policies and logic.
Entrenching Biases
Without vigilance, the reliance on biased datasets cements discrimination into systems impacting people's opportunities and lives. Proactively identifying and mitigating unfair biases is critical.
Safety and Control Risks
If granted physical agency like with robotics or automation, brittleness and unpredictable behavior pose physical dangers without extremely rigorous testing and validation. Human control must remain present.
Spread of Misinformation
The difficulty verifying reasoning in opaque AI systems enables the spread of misinformation when deployed irresponsibly or with malicious intent. Strict accuracy standards are essential.
Current Initiatives to Overcome AI's Limitations
While significant obstacles remain, researchers globally are exploring strategies to address the fundamental weaknesses of AI, including:
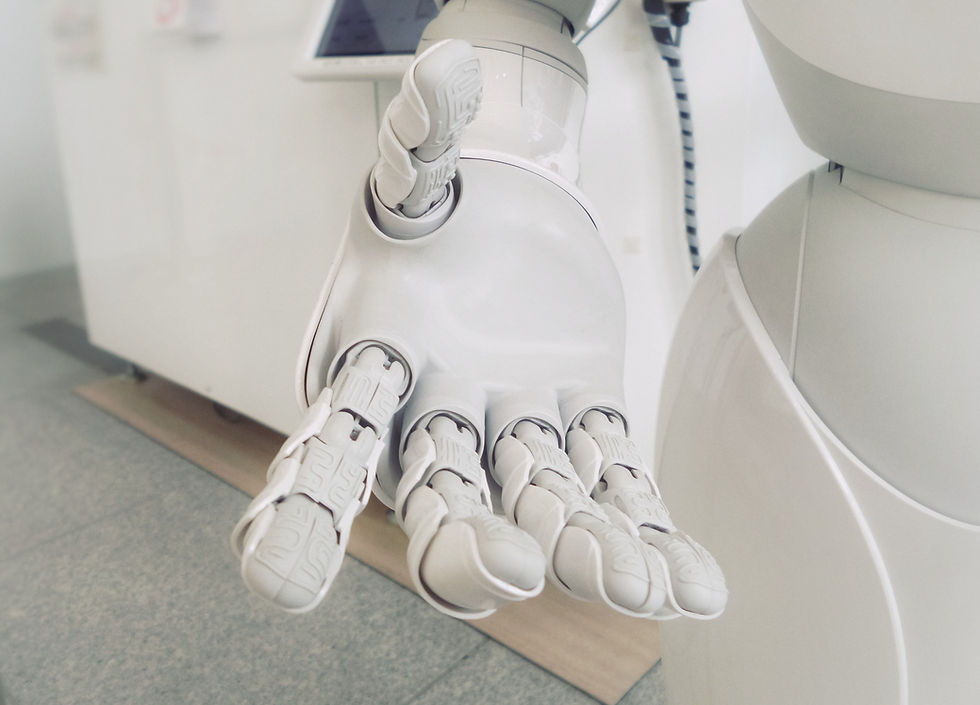
Hybrid AI-Human Collaboration
Rather than full automation, human-AI collaboration allows people to handle complex reasoning and nuanced judgment, while AI contributes to speed, precision, and processing scale beyond human capability.
Explainable AI (XAI)
XAI focuses on surfacing an AI's internal logic and making it more interpretable, transparent, and accountable to users. This ensures suitability and avoids blind acceptance of AI behaviour.
Transfer Learning
Transfer learning aims to enable applying knowledge across tasks, avoiding retraining from scratch each time. This more closely resembles flexible human learning.
Lifelong/Continual Learning
These training approaches aim to mimic biological learning, expanding knowledge continually without catastrophically forgetting previous learning.
Adversarial Robustness
Making AI algorithms more resilient to minor input variations or external noise will reduce brittleness and unforeseen edge cases. This improves real-world functionality.
Trusted AI Pipeline
Governance frameworks focusing on data quality, transparency, explainability, fairness, and security aim to build trusted, responsible AI from the start of the pipeline.
General AI
Hypothetical future AI possessing more generalized intelligence across domains, not bound to narrow specialities, promises to overcome many limitations like brittleness, opacity, and lack of reasoning. But achieving general AI remains highly speculative.
Practical Implications of Current AI Limitations
Until fundamental progress toward general AI is made, enthusiasts must set realistic expectations and use caution when applying today's limited AI technology. Some implications to inform responsible use:
Avoid Full Automation
Where feasible, keep humans in the loop rather than handing full agency over to an algorithm. Ongoing human auditing helps catch inevitable AI errors or misjudgments.
Establish Clear Accountability
Liability frameworks should designate who bears responsibility when AI systems err or cause harm - whether developers, users, or companies employing the AI.
Isolate from Critical Systems
Brittle or opaque AI algorithms should not be integrated into systems like medical devices or transport vehicles where failures pose a significant public danger. Isolation reduces risks.
Use AI Probabilistically
Rather than absolute reliance, probabilistic AI outputs indicating confidence percentages allow more nuanced human interpretation and discretion to override uncertain AI judgments before acting.
Employ Ensembles
Using diverse ensembles of multiple AI models hedges against individual model weaknesses. More holistic judgment arises from reconciling ensemble variation into consensus.
Verify Sensitive Decisions
For weighty predictions like loan approvals impacting people’s lives, critical manual auditing and verification of fair justification must occur, reviewing factors the AI deemed relevant.
Monitor for Fairness
Check labels and performance across sensitive subgroups in the training data to verify the AI treats all groups equitably. Remediate unfair correlations.
Extensively Test Edge Cases
Expose the trained system to a wide range of stress tests and adversarial examples to probe where it breaks and ensure generalization within acceptable bounds.
The Future Path of AI
While today's AI limitations warrant measured expectations, the technology continues rapid evolution. Some promising directions include:
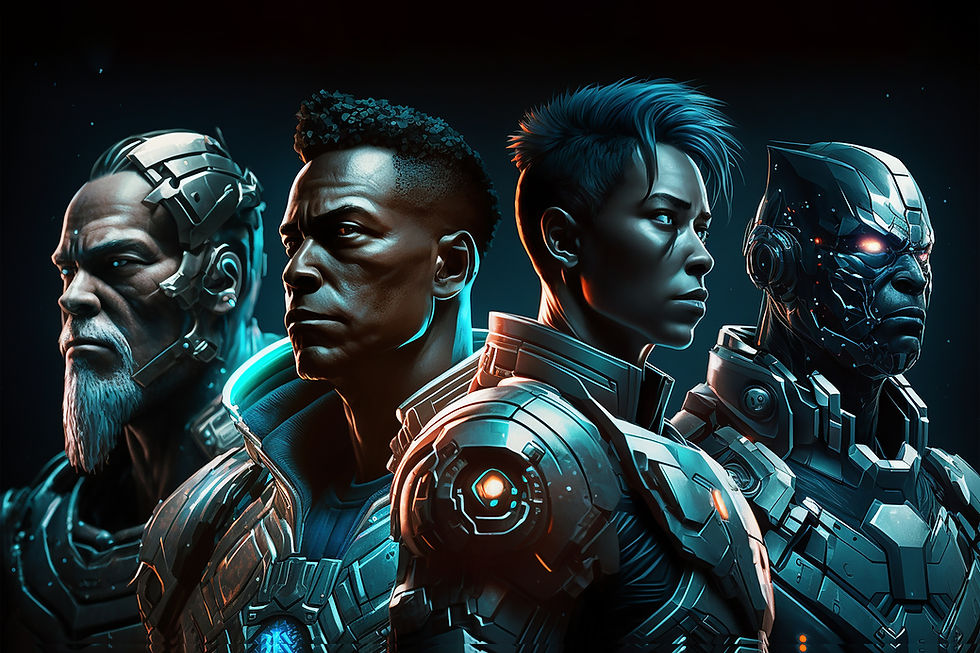
Integration of Reasoning, Logic and Knowledge
Equipping AI with more capacities like reasoning, abstraction, causality, and contextual knowledge representation will reduce brittleness and increase rationality.
Multimodal AI
Combining distinct modalities like vision, language, speech, motor skills and cognition allows more human-like awareness and versatility within AI systems.
New Fundamental Techniques
Architectures beyond today's predominant neural networks may prove superior for expanding capabilities. Hybrid neuro-symbolic, topological, liquid state machines and quantum computing approaches show promise.
Improved Generalization
Techniques that help AI learn expansive, abstract patterns and representations will improve generalization beyond specific training data.
Richer World Models
Knowledge graphs, physics engines, and other methods to endow AIs with general world knowledge will aid more flexible reasoning and reduce blind spots.
Plasticity
Enabling ongoing malleable learning without catastrophically forgetting will allow AIs to adaptively expand skills throughout their lifespan like biological brains.
Artificial Intelligence (AI) Tools that Can Improve Your Productivity
Staying productive is crucial but increasingly challenging. Luckily, artificial intelligence (AI) tools can help streamline workflows and automate tedious tasks. In this section, we'll explore some of the top AI-powered productivity tools on the market right now across these key categories:
Creating Videos
These can help you quickly create professional videos without technical expertise.
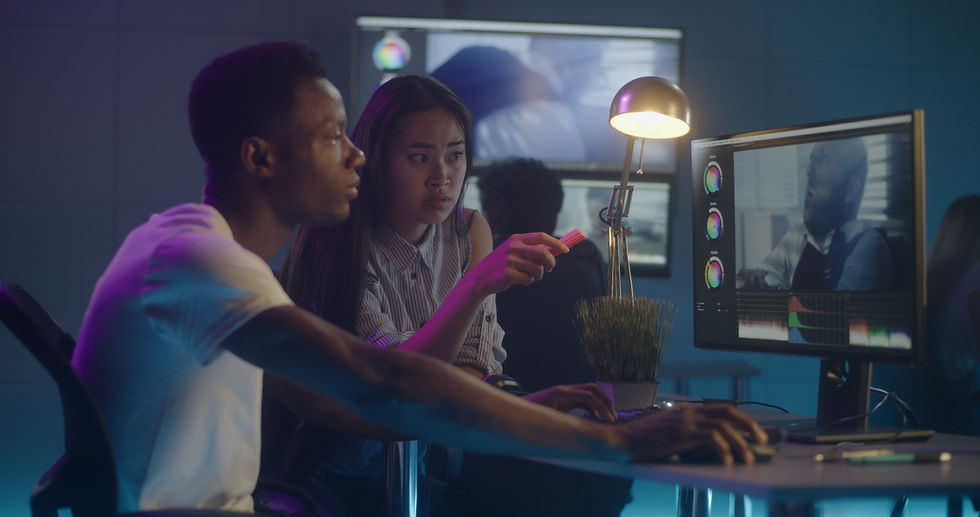
We have also discussed the following topics in other articles:
Podcast Production
Tools like Podcastle AI and Fliki make it easy to automate podcast editing and publishing.

Website Building
Platforms such as Elementor allow you to build sites through an intuitive drag-and-drop interface.
AI Article Writers
Services including
Kafkai
GetGenie can generate blog posts and articles from prompts.
Take a look at other articles we have written about Scalenut:

Email Outreach
Presentation Building
Desktopus can turn text into polished slide decks in just minutes.

By incorporating these AI tools into your workflow, you can work smarter, faster, and more efficiently. Let your productivity reach new heights with artificial intelligence.
Final Thought: AI Limitations
Current artificial intelligence has incredible strengths like statistical pattern recognition and computation at an inhuman scale.
However, broad limitations around reasoning, common sense, brittleness, and flexibility constrain today's systems. Appreciating these current restrictions allows appropriately cautious adoption while the technology continues rapidly evolving.
With a balanced understanding of its weaknesses and realistic expectations about use cases, we can harness AI’s immense current capabilities while pursuing new techniques that overcome limitations and unlock the full transformational potential of artificial general intelligence in the future.
You Can Also Read:
10 Reasons Why Bluehost is the Best Web Hosting Provider for Your Business
Hostinger Webmail: A Reliable, Secure and User-Friendly Email Platform
Unlock the Full Potential of Your Video-Creation With Pictory.ai's Lifetime Deal
Maximize Your Website's Performance With Hostinger Login's Advanced Features
Maximize your earnings: How to Start Affiliate Marketing With No Money
Maximizing Your GetResponse Login: Tips and Tricks for Increased Productivity
How HercuList Plus Can Help Small Businesses Advertise More Efficiently
Step-by-Step Guide to NordVPN Login: Access Your Account in Minutes
How to Use NordVPN to Bypass Censorship and Geo-Restrictions
What are The Benefits of Using Pictory AI for Social Media Marketing
How Pictory AI's Machine Learning Algorithms Make Video Creation Easy
10 Reasons Why Systeme.io is the Best Platform for Online Entrepreneurs
What are the Frequently Asked Questions (FAQs) About Scalenut?
Comentarios